With ChatGPT, AI has officially arrived. It was a buzzword before, now it’s an everyday, in-your-face, job-changing reality that is impossible to ignore.
We’ve come to a point, in fact, where the faceless robot overlords who rule our lives seem omnipotent. So people are starting to think that the machine learning and predictive analytics of AI can tackle any mystery.
Like identifying which team will win the World Series.
Right?
Unfortunately for every sports bettor in Vegas, AI isn’t reliable when it comes to those kinds of predictions.
(Cue sad trombone.)
Why not?
Many people think of AI as a panacea, a point-and-shoot solution to every question. And indeed, AI can unlock a tremendous amount of information that helps us make sense of our world.
But when it comes to making sense of the World Series, AI is as clueless as the rest of us.
Because baseball – like most sports – is a stochastic process. And AI isn’t competent at understanding random patterns.
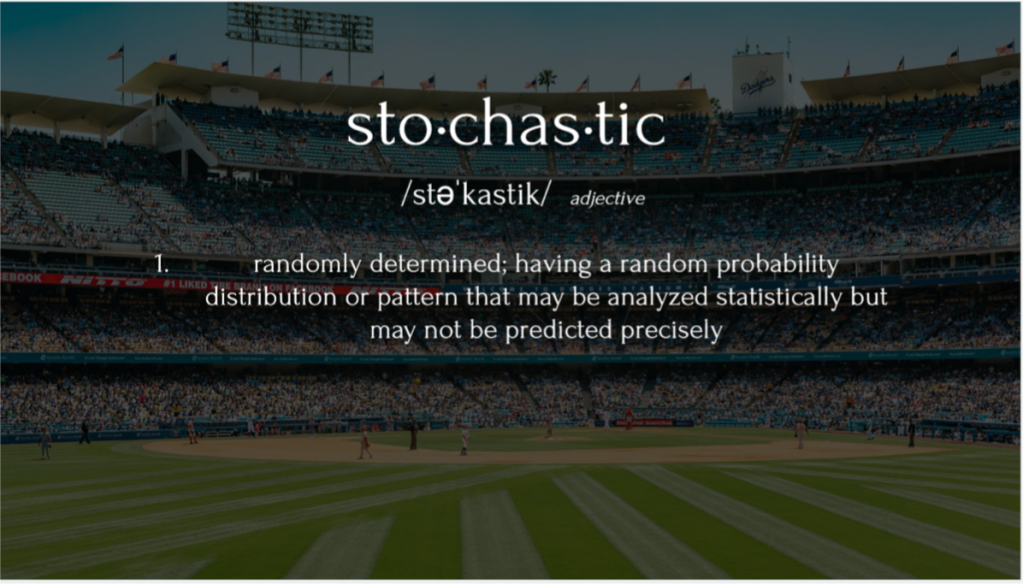
People may think that baseball teams are – at any given period – somewhat predictable. But this is far from true.
The game is, in fact, all about random variables.
Perhaps a player is injured. Another player is on a streak. A different player throws the game. This element of chance means that mutations intrude upon patterns.
AI doesn’t like that.
Machine learning needs similar environments to be successful. The phenomena in a training environment must be the same in the real world.
But what about “Moneyball” and the whole sabermetrics angle, you ask?
If Brad Pitt can predict baseball, why can’t the robots?
Moneyball is not AI; it’s statistics. And stat models are very different from AI models.
ALSO READ:
WITH PHYSICIAN RECRUITING IN DIRE STRAITS, CMOS ARE EMBRACING A NEW KIND OF MONEYBALL
Stat models are built on assumptions. There is a known outcome that you’re trying to arrive at. You’re applying rationality to the process.
For example, your model can assume that normally, you expect a certain batting average from a particular player. It’s never below or above a certain number. Your goal is to box in your assumptions.
AI models, on the other hand, are assumption-free. You’re simply extracting data, not trying to push your beliefs on the machine or algorithm.
AI doesn’t care if you’re working in a linear environment. The machine arrives at its own values.
The stat model is a static snapshot of the world at one moment in time. And it’s usually built by hand. The AI model is more nimble and applicable to a real-world setting.
Sports analytics is very popular now. It’s so nascent that people are trying to figure out what, exactly, they should be figuring out.
In the original Moneyball experience, the objective was to get the right people and put them in the right places to win games.
Now the moneyballers of the world are thinking through whether analytics is best applied to winning games…
…Or best applied to who should be on the teams…
…Or another angle.
Wherever moneyball may be headed, it’s got nothing to do with AI.
WHY BRAIN MACHINE INTERFACES BUT NOT BASEBALL?
Here’s what seems to be confounding about AI:
Predicting the World Series is too hard for the machine; So why are other seemingly impossible things easy for AI?
Like…literally read our minds.
A cutting-edge technology called brain machine interfaces (BMIs) will allow us to communicate via thoughts, opening groundbreaking new doors in the realms of communication, collaboration, and creativity. Still in its early stages, the BMI industry has been making exponential strides towards a world in which most humans will have a device safely implanted in their heads.
Seems crazy – but not so for Elon Musk, who is developing BMIs through his company Neuralink.
So this disparate ability with AI seems confusing at first. Shouldn’t it be easier to predict baseball than interpret human thought?
Well, if baseball is less predictable than you think, it turns out that human behavior is more predictable than you might suspect. And this is all because of heuristics.
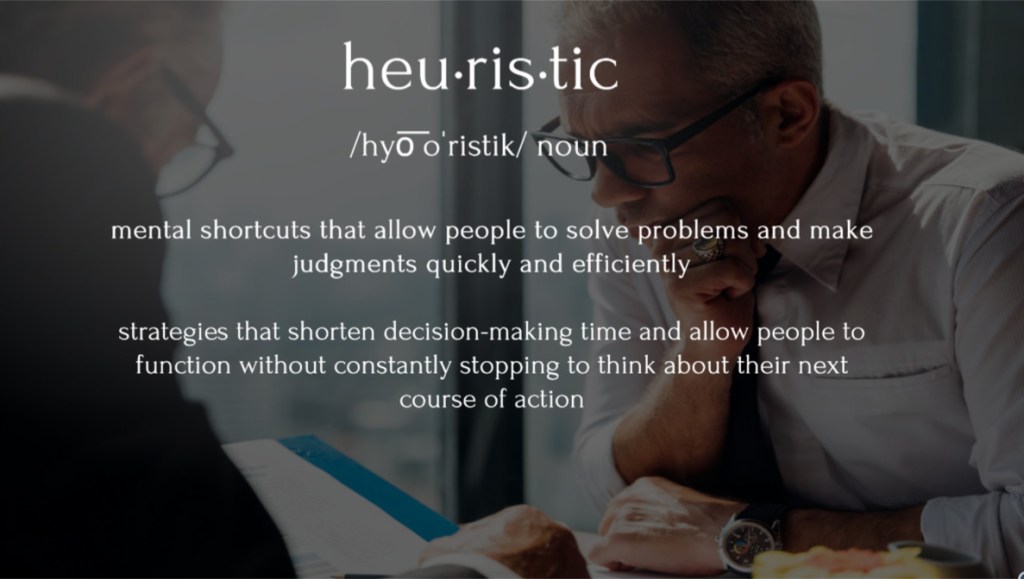
Heuristics are the strategies we create based on our previous experiences with similar problems. They’re a process that humans use to make decisions.
But humans can only handle two to three variables with heuristic processing. The AI machine can handle 20,000.
So the machine knows better than you do about how you’re going to respond to different circumstances. It knows how you’re going to think.
Again, crazy. But this is exactly where AI shines.
People believe that they’re one of a kind. You think you’re special – and to your loved ones, you absolutely are.
But to the machine, not so much. Because you, dear reader, are human. And – even if you don’t think you do – you march to the drumbeat of crowd mentality.
Many AI applications play those odds. They specialize in how they look at people, and as such, AI can operate with high statistical confidence regarding how you will think next.
Humans can’t see the patterns that reveal predictive behavior. But machines can.
It’s a shame that those same machines just can’t seem to interpret how nine Diamondbacks players will handle a baseball.
Seems so simple. But the good news is that maybe there are some areas where humans are still smarter than machines.
You can bet on that – even if AI won’t help you actually win on that bet.